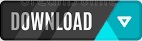
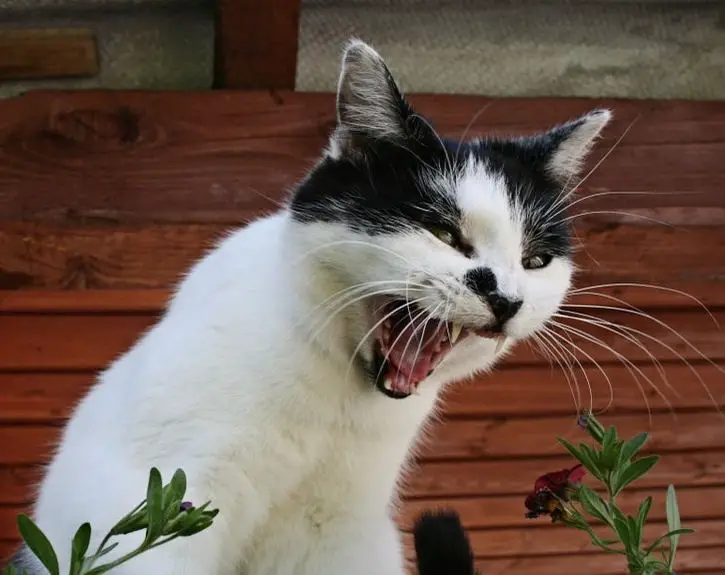
Therefore, if the features are properly segmented in multiple frequency bands, we can expect the better classification performance. The log power spectrogram and mel spectrogram of the sample data taken from each class of CatSound dataset, as illustrated in Figure 4 and Figure 5, indicate that the activities of the cat sound in frequency bands are different depending on the sound class. This is an effective way to deal with frequency varying data, such as cat sounds, in which the frequency components in a certain band are active for some specific sound classes. This is an area specific feature extraction, where we first divide the feature map into low and high frequency bands and then apply the GAP in each band. In this work, we used frequency division average pooling, which is modified version of GAP. The pictorial representation helps the reader to understand nature, amplitudes and time duration of the sounds. The waveform representations of 10 samples from each class are visualized in Figure 2. Even in some classes of cat sound, the data may have varying length because the bio-diversity widely differs depending on geographical locations, cat species, and ages. On the other hand, the cat sounds in rest (“ purring”), warning (“ growling”), mating (“ gay-gay-gay”), fighting (“ nyaaan”), angry (“ momo-mooh”), and want-to-hunt (“ trilling or chatting”) are usually more meaningful if they are analyzed in longer time duration. For example, the sound of the cat in normal mood (“ meow-meow”), defensing (“ hissing”), a kitten calling its mother (“ pilling”), and cats in pain (“ miyoou”) can be semantically correct, even in short time duration. Some audio files in our dataset have been divided into several segments with varying length, each of which has the same semantic category in the same way used in for music information retrieval.
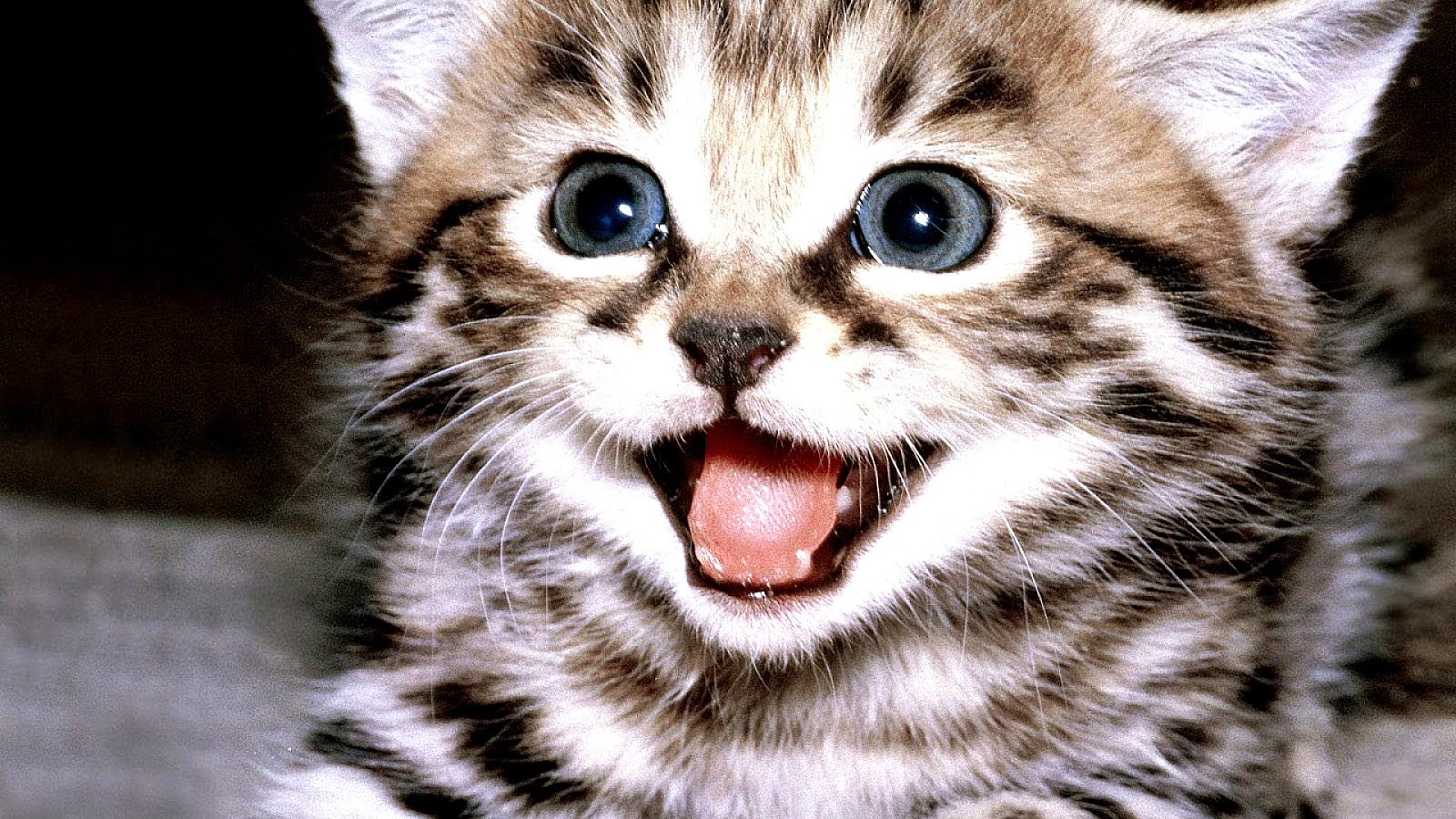
Therefore, with the combination of all those positive factors, we obtained the best result of 91.13% in accuracy, 0.91 in f1-score, and 0.995 in area under the curve (AUC) score.
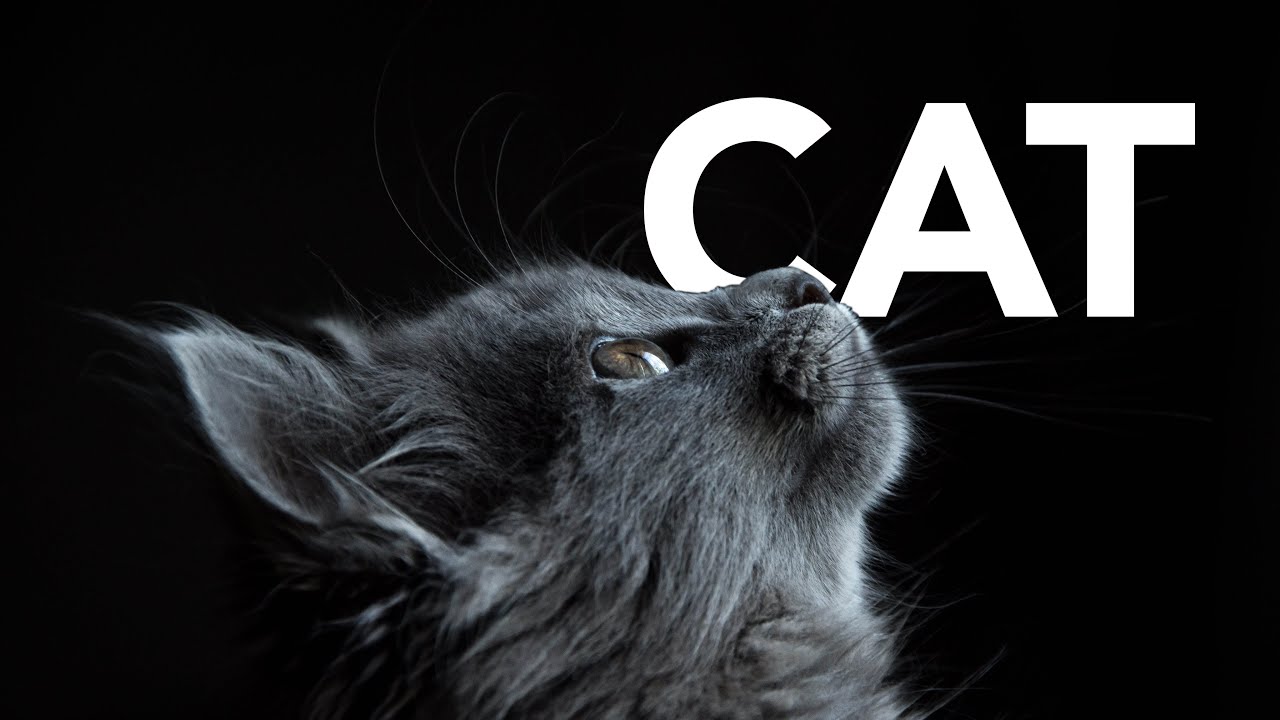
Moreover, both learned features from pre-trained CNN and unsupervised CDBN produce good results in the experiment. As expected, the proposed FDAP features with larger amount of data increased by augmentation combined with the ensemble approach have produced the best accuracy. We compare the classification performances with respect following factors: the amount of data increased by augmentation, the learned features from pre-trained CNN or unsupervised CDBN, conventional GAP or FDAP, and the machine learning algorithms used for the classification. For the classification, we exploited five different machine learning algorithms and an ensemble of them. In FDAP, the frequency dimension is roughly divided and then the average pooling is applied in each division. In addition to conventional GAP, we propose an effective pooling method called FDAP to explore a number of meaningful features. In this study, we use two types of learned features from deep neural networks one from a pre-trained convolutional neural net (CNN) on music data by transfer learning and the other from unsupervised convolutional deep belief network that is (CDBN) solely trained on a collected set of cat sounds. Along with the original dataset, we increase the amount of data with various audio data augmentation methods to help our classification task. Machine learning approach for the classification requires class labeled data, so our work starts with building a small dataset named CatSound across 10 categories. In this paper, we deal with the automatic classification of cat sounds using machine learning. The domestic cat ( Feliscatus) is one of the most attractive pets in the world, and it generates mysterious kinds of sound according to its mood and situation.
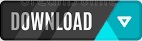